At Violet, we have a strong belief that the systems we operate within are not built equitably for all people. This is particularly evident in health care, shown in the many health disparities that affect women, BIPOC and LGBTQIA+ communities, veterans, and more.
To begin combatting a broken health system, we created a standard measure of cultural competence within providers called Violet Benchmarks—a measure that allows for a shared, objective understanding of quality of care, particularly for BIPOC and LGBTQIA+ patients. Over the last two years, we’ve worked hard to build, test, and continuously refine Violet Benchmarks to maximize better health outcomes for patients.
The Benchmarks use an algorithm that takes into account various sources of provider data, amongst which are self-attested by providers as well as third-party claims data. As we worked with claims data to determine how best to enhance our algorithm, our researchers noticed some glaring problems.
In this series of investigations, we are taking a deeper look into routine diagnostic coding practices to uncover health inequities occuring in BIPOC communities.
Looking at the data.
To begin this process, our team used claims data to create community models which could approximate demographic data for provider patient panels.
Annie McKinnon, a senior data scientist here at Violet, built the demographics model to approximate what percentage BIPOC patients accounted for in an individual provider’s patient panel. But in the process of doing so, it became shockingly clear that there were disparities in the diagnosis and coding percentages.
“When I initially produced this data set, I was looking for another way of validating some of the community demographics models we would use to benchmark providers,” she explains. “Knowing that some conditions are more prevalent in certain populations, I was hoping to see that borne out in the data for a few examples of those conditions.”
We are already aware of the myriad of health disparities affecting BIPOC communities, as they are well known to anyone who is invested in health equity. However, we didn’t expect to find the coding discrepancies as starkly present when we depended on patient race as an aggregate. What we found buried beneath the more obvious disparities was also an insidiousness to coding practices that produced these discrepancies. Annie explains further what happened:
One of the first things I looked at was the diagnosis codes that were most prevalent in the Black population. I sorted the diagnosis codes to show where Black patients made up an outsized percentage of who that code was assigned to. As I suspected, sickle cell anemia was among the top codes based on this sorting (22nd highest) but above that code were also a few unexpected codes: Z7142 - Counseling for family member of alcoholic (1st), Z7152 - Counseling for family member of drug abuser (2nd), and R461 - Bizarre personal appearance (4th). It quickly became clear to me that in addition to seeing conditions that are more prevalent in specific populations, this was showing bias in our medical system and other structural biases in our world. While my expertise extends to aspects of data and not clinical ones and thus limits the set of conclusions I might draw, I am left with a lot more questions about these biases.
It quickly became clear to me that in addition to seeing conditions that are more prevalent in specific populations, this was showing bias in our medical system and other structural biases in our world.
Documenting health disparities in Black and AAPI patients.
Of the entire US patient population, Black patients make up 16.1%, and Asian American and Pacific Islander (AAPI) patients make up 8.6% and white patients make up the vast majority at 61.2%. Despite making up a significantly smaller number of the overall patient population, there were instances where Black and AAPI patients had double or nearly triple the percentage of certain diagnosis, compared to white patients.
We created the following chart based on US Census race categories, with the highlighted numbers illustrating the percentages of a specific race from the total patient population with the corresponding ICD-10 or CPT code.
ICD-10 and CPT codes overrepresented in Black patients
.png)
The above chart shows how heart-related disorders, diagnosis around maternal and fetal health, and conditions related to sickle cell anemia are all disproportionately higher for Black patients than the patient population (16.1%) and disproportionately smaller for white patients compared to the patient population (61.2%).
These percentages drive home the stark statistics already seen in the Black maternal health crisis, the effect of racism on sickle cell disease and related conditions, and racial disparities surrounding coronary artery disease. In each of these cases, it is not a person’s race that is a determinant of their health in any biological sense, it is racism—structural, institutional, and interpersonal—that is the true cause of poor outcomes.
ICD-10 and CPT codes overrepresented in AAPI patients
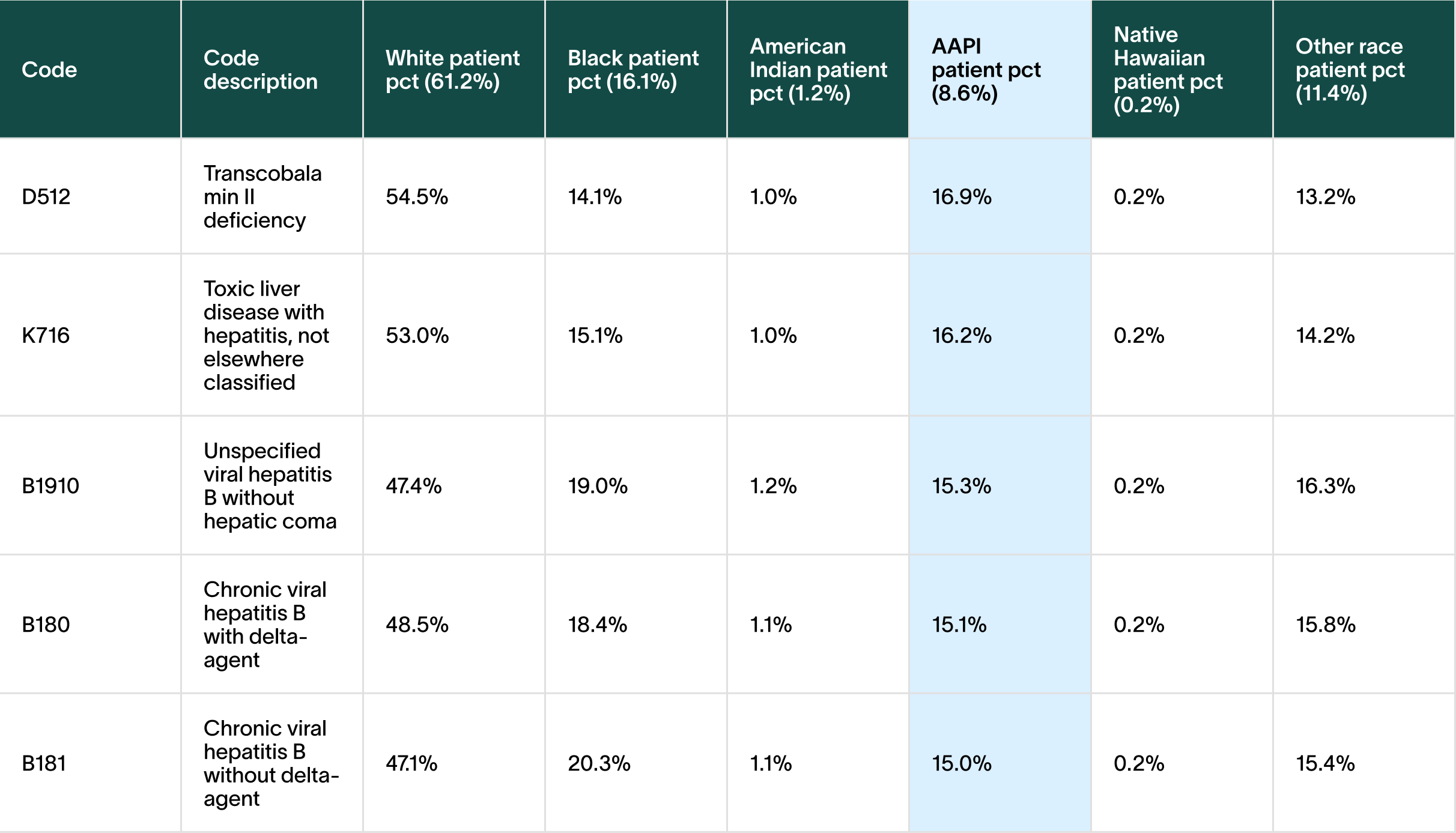
For AAPI patients, diagnoses around Transcobalamin II deficiency (vitamin B12 deficiency) and Hepatitis B were nearly twice as high as the patient population (8.6%). According to the CDC, Asian Americans account for 58% of the 862,000 Americans living with chronic hepatitis B, as countries in Asia and the Pacific Islands have medium to high levels of hepatitis B. Some studies have shown that B12 deficiency has a higher prevalence in people of South Asian descent, with one theory pointing to vegetarianism and dietary patterns as a cause. [Source, source]
Uncovering coding discrepancies for Black and AAPI patients.
After looking at the health disparities present in the data, it also became apparent that there were codes attributed to Black and AAPI at significantly higher rates than white patients.
Among the discrepancies found, Black patients accounted for 32% of the code, “bizarre personal appearance” (nearly twice the 16.1% patient population), 28% of “need for continuous supervision”, 28% of “illiteracy and low-level literacy”, 23% of “mixed receptive-expressive language disorder”, and 22% of “social pragmatic communication disorder”.
ICD-10 and CPT coding discrepancies for Black patients
The highlighted numbers illustrate the percentages of Black patients with the corresponding ICD-10 or CPT code from the total patient population.
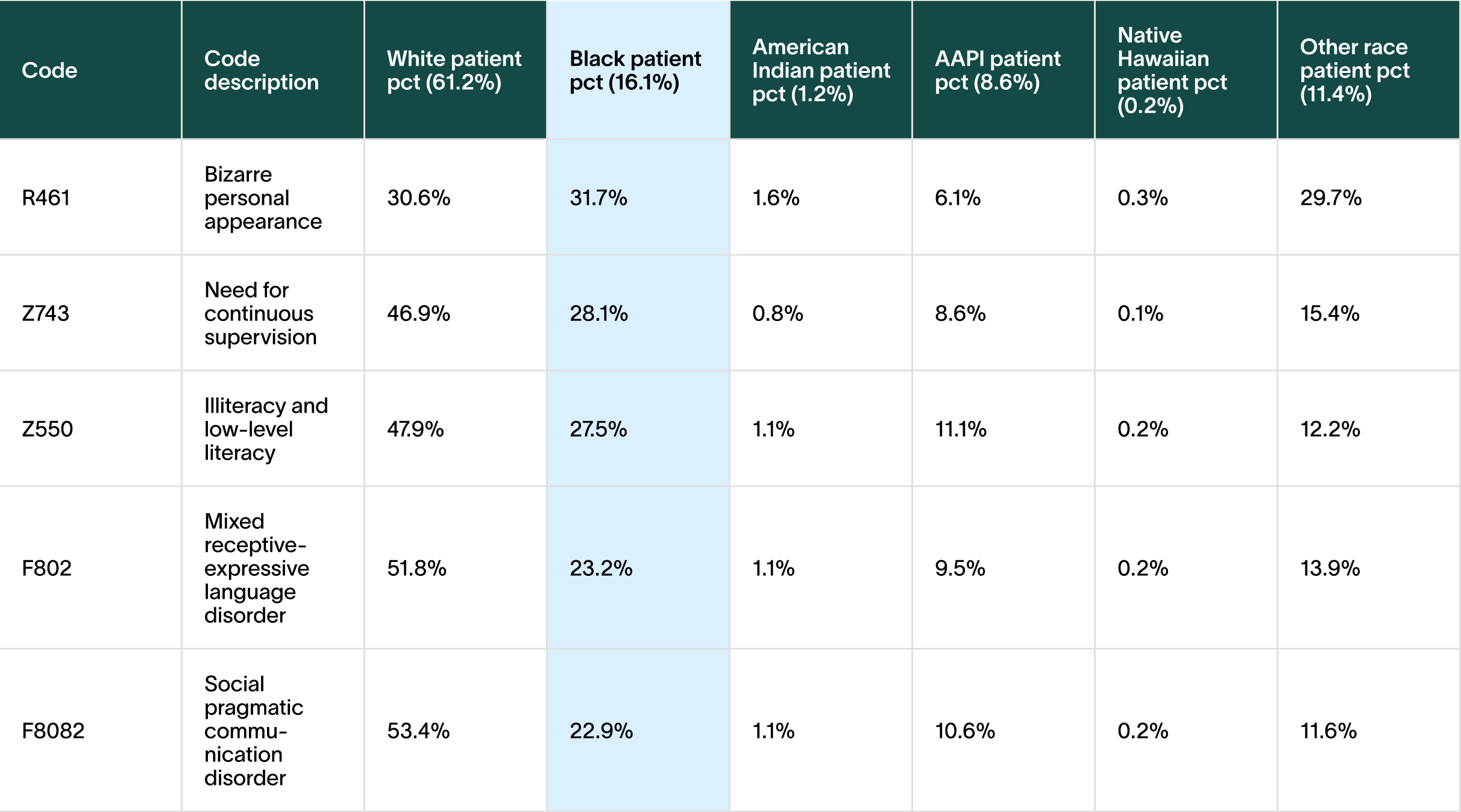
Why do BIPOC patients have these codes attributed to them at disproportionate rates?
Our hypothesis behind the use of codes like “bizarre personal appearance” and “need for continuous supervision” is due to doctors overpathologizing Black patients, or in other cases, attributing a negative bias they wouldn’t otherwise apply to white patients. (We plan to discuss this in more detail in Part 2 of this series.) Literacy, language, and communication related coding discrepancies in the Black patient population can be also attributed to the bias of misattributing language differences as language disorders—particularly in Black children.
ICD-10 and CPT coding discrepancies for AAPI patients

For AAPI patients, the code for “encounter for childbirth instruction” was nearly triple the population size—but why is this code so high for AAPI patients in particular? Our hypothesis is that clinicians are not trusting AAPI patients to do correct childbirth processes either because of the prevalence of non-allopathic medicine practices in APPI cultures, or because clinicians are assuming these patients don’t know the “right” childbirth practices in terms of Western medicine.
The coding for “worries” was more than double the population size of AAPI patients. Since these encounters occurred in a primary care setting, we suspect that it’s related to the misconception that AAPI patients somaticize their emotional symptoms. Some doctors believe that if a patient presents with symptoms that don’t seem to have a cause, it’s a result of worry. Alternatively, there’s a commonly held belief that AAPI patients won’t go to mental health providers and instead will go to a primary care provider.
For immunization contraindication, our hypothesis is that it’s patients using non-allopathic medicine, and the result of clinicians not having knowledge of or knowing how to work with traditional medicine when combined with Western allopathic medicine.
What does this mean?
When reflecting on initially producing this data, Annie explains, “I approached this from the narrow perspective of validating some of our assumptions around benchmarking providers, but our definitive data is a very rich data set and we can see correlation with conditions and behaviors both in the data itself, especially so when combining it with other data sources such as the American Community Survey. I hope we continue to have the opportunity to explore this data, improve our provider badging models, and expose aspects of how the medical system is impacting specific communities.”
Our standardized measure of cultural competence was created with the goal of improving patient outcomes. As we continue to hone and refine Violet Benchmarks, it’s imperative that we uncover the biases that drive these coding discrepancies, as well as the systemic issues causing health disparities within BIPOC communities.
This is part one of a three-part series of thought leadership from Violet.